Seclea Platform makes black-box AI transparent and clearly explain its decisions, behaviour and evolution-overtime. Enabling the data science team to focus on the critical tasks of building robust and high-performance AI algorithms.
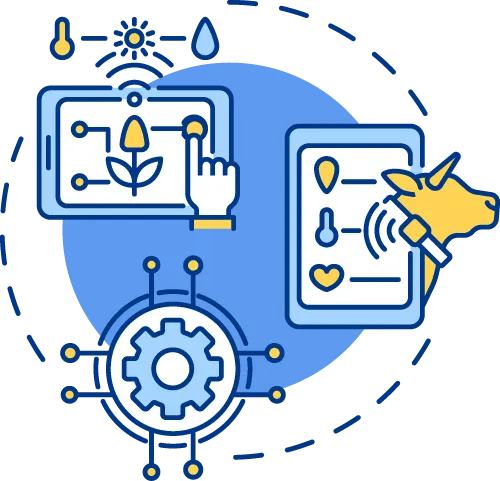
Decision-Level Explanation
Explaining with context and factors that played a critical role in individual decisions.
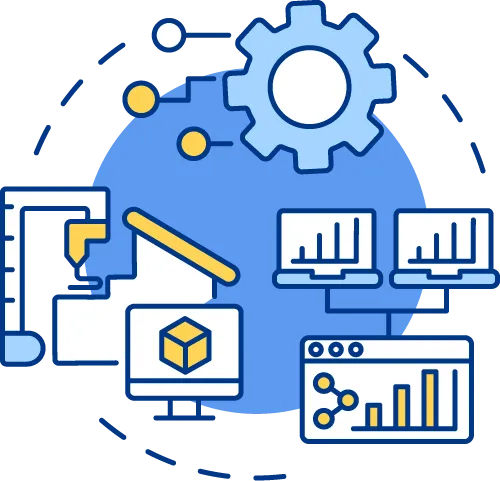
Model-Level Explanation
Understand the model behaviour in the context of its decisions and time.
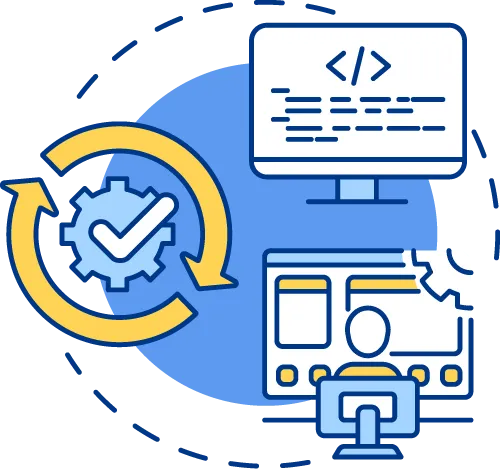
Causation-Level Explanation
Explain the evolution of a model and trace back decisions to machine and human actions.
Decision-Level Explanation
Machine learning and deep learning models process input data to generate output, aka decisions. For every decision, the two critical elements are the input data and the internal dynamics of a given model.
A decision-level explanation explains how the internal dynamics interact to reach a decision. The decision explanation has a specific scope for the subset of the internal dynamics that correlate a given data input-decision relationship. This explanation provides quick reasoning for what input parameters were essential to a model in reaching the decision.
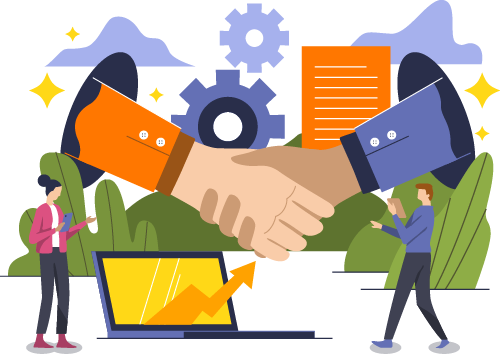
Model-Level Explanation
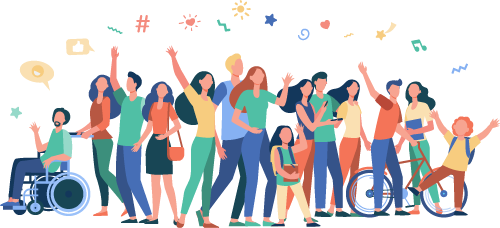
Model-level explanation helps understand the overall behaviour of a machine learning or deep learning model. A model in the testing or deployment makes numerous decisions based on the varying inputs. Understanding how the variations in input data to decisions are evolving help track the behaviour of the model in both the context (input data variations) and time.
Understanding a models’ behaviour provides a more comprehensive picture of how the internal dynamics are interacting – over a set of input data and time—providing a better understanding of a model and ensuring it is fair, transparent and performs within set safety limits.
Causation-Level Explanation
Understanding the interactions of internal dynamics of a machine learning or deep learning model is essential. However, this does not explain how the models evolved these internal dynamics and their relationships or dependencies over time.
Causation-level explanation describes the evolution of a model that impacts its behaviours and decisions. Model evolution combines the influences of datasets, model design/development, and input-data/decisions on the internal dynamics that led the model to its current state—providing full traceability of a decision and model. An essential feature for AI accountability, auditability and transparency.
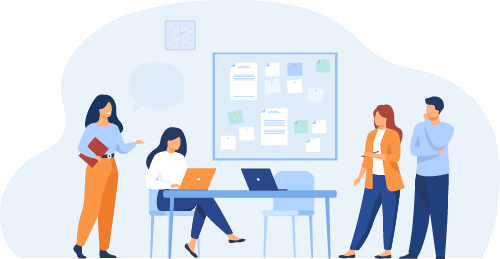
Why Seclea Explainable AI?
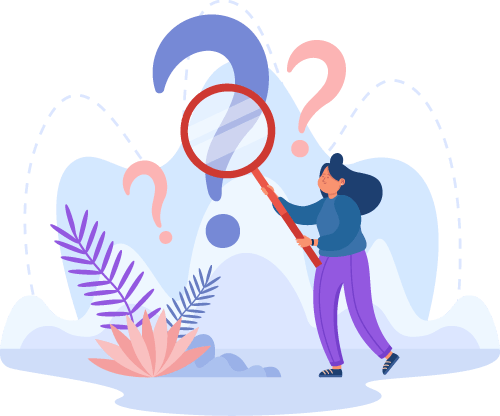
Seclea explainable AI provides you with three levels of explanations. You want to understand why a decision was made; the decision-level explanation offers the answer.
You want to understand and report the behaviour of a model; the model-level explanation sketches the whole picture for you.
Suppose you want to know why a model evolved to make such decisions or current behaviour. In that case, the causation-level explanation helps you understand a models history and various influences that shaped it.
Seclea Platform enables transparent and explainable AI applications with full traceability. Ensuring you gain the trust of all stakeholders and regulatory compliance.
How Does Seclea Works?
Seclea Platform easily integrates with your existing AI development pipelines or deploy applications. We support a wide range of machine learning and deep learning algorithms, so you focus on having the best solution for your business challenge. And leave the explanation of your AI applications and their decisions to Seclea.
Why don’t you take Seclea Platform for a test drive?
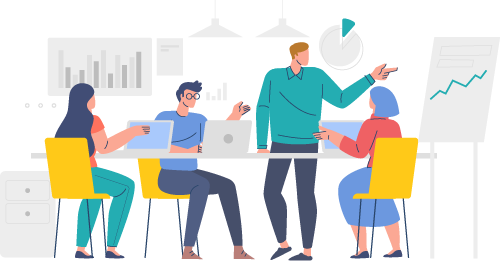