Artificial Intelligence (AI) can potentially help reduce human error, increase productivity and make a world more efficient. AI is becoming increasingly used to make decisions such as approval of a loan, screening resumes, and disease diagnosis. Such decisions on time are crucial and beneficial to individuals. However, if these discriminate against anyone based on ethnicity, gender, age, or other characteristics, AI can be detrimental to individuals and society. Such unfairness and discrimination are termed AI bias, which can be defined as the inclination or prejudice exhibited by AI decisions for or against one person or group, especially in a way considered unfair.
AI bias can be present or introduced at any stage of the AI lifecycle. The core reason we have AI bias is the conscious or unconscious biases in humans, which are then transferred to machines via data, algorithm design & development, and how AI is used. Our society, unfortunately, is full of prejudices that may be reinforced in data or model design. We need to make sure that we understand AI biases and when and where to be cognisant of them. Only with a careful understanding of AI bias, a collection of diverse inputs from the broader community into an AI application’s design & development and continuous validation during the entire lifecycle can we be confident to a certain degree that the respective AI application won’t have any fairness issues.
So, let’s look at a few most common types of biases, such as:
Selection bias: The model is created by selecting particular types of instances more than others. For example, a face-recognition algorithm may have more photos of light-skinned faces than dark-skinned faces in its training dataset. This potentially leads to poor performance in recognising darker-skinned faces.
Algorithm bias: When there are unrepresentative datasets, inadequate models, weak algorithm designs, or historical human biases can result in unfair outcomes. For example, Facebook’s advertisement placement algorithm showed career advertisements more frequently to males over females despite initially aiming to be gender-neutral in targeting prospective job candidates.
Measurement Bias: This bias occurs when data collected differs from reality due to inconsistent features or labels used during the prediction. Furthermore, such measurement bias could lead to a negative impact with distorted outcomes. For example, cameras that capture images may have different brightness filters, leading to false ideas and biased results.
Exclusion bias: Exclusion bias happens in data pre-processing by removing some features, thinking that they are irrelevant. Unconscious prejudices that the individuals who design the algorithms hold can cause the removal of specific data unrelated to that person. For example, suppose a company opens a vacancy for students from the top 20 best universities in the UK and excludes those students from the university based on their previous performance records. Such exclusion could lead to unintended bias in the universities’ acceptance criteria.
Out of all, one of the most common forms of bias in AI is gender bias. Unfortunately, instances of AI models exhibiting gender bias are evident across many applications, including recruitment, credit card approvals, healthcare, etc. Below are some examples of gender bias identified in major AI applications:
- 2018, Google Translate was shown to have a gender bias when translating from gender-neutral Turkish to English.
- 2019, several complaints were made regarding Apple’s credit card that the application approval algorithm unintentionally made discriminatory decisions against women.
- 2020, A documentary by Joy Buolamwini discovered that existing AI facial recognition systems do not identify dark-skinned and female faces accurately.
- 2021, A study published in Nature on sex and gender differences and biases in AI for biomedicine and healthcare states, “AI technologies do not account for bias detection. Most algorithms ignore the sex and gender dimension and its contribution to health and disease differences among individuals”.
One of the reasons for gender bias in AI is the lack of diverse representation in data. This can have a diverse range of negative impacts. It can create an inaccurate picture, concealing vital differences between people of a different gender, which are most apparent and impactful in healthcare. It can also lower accuracy on these under-represented groups as the model has less data to learn the genuine relationships present in the data. Women often have less formal financial history recorded due to their lower participation rate in employment and other factors.
Having enough representative data doesn’t’ always solve the problem; even when the data perfectly represent reality, this can end up embedding existing societal biases in the AI application. For example, globally, women’s income is often less than men’s even for the same job level, creating a skewed income profile in the dataset, which can impact the AI that may use that to decide to give a lower credit limit to women.
AI bias has real consequences; this problem has already caused physical, mental, and financial issues for women who cannot get fair and equal treatment in healthcare, credit cards, mortgages, and jobs.
To create a fair and unified society, it is crucial to ensure that AI is not biased towards a particular subgroup within a population. The following steps need to be considered.
Diversity in the data: Ensure the training dataset represents individuals and subgroups fairly. Data is the foundation of any AI application, so accessing datasets for representation from different gender and identifying the gap. Further, using a gender lens while collecting and analysing data can ensure diversity in data.
Explainable AI: Implement explainable algorithms that provide understandable explanations for the layperson. Explainable AI can highlight any potential bias in the decision. For example, Explainable AI might highlight that a female is given less credit because of her gender – indicating the unwanted influence of gender on the decisions.
Integrated bias detection: For every step of the AI lifecycle, organisations should have an integrated bias detection and mitigation system in place. Organisations should ensure that bias, even in the corner cases, will be identified pre-market and post-market phases of an AI application.
Innovative techniques: Develop Machine Learning algorithms that have integrated de-biasing techniques, which offer ways to penalise errors in recognising the primary variable and have additional penalties for producing unfairness.
Gender-neutral language: Introducing gender-neutral languages can also prevent gender bias in AI language models. Even as recent studies have sought to remove bias from learning algorithms, they largely ignore decades of research on how gender ideology is embedded in language.
Policies in the company: Organisations need to establish guidelines, rules, and procedures for identifying, communicating, and potentially mitigating gender bias. The study shows that diverse demographic groups are better at decreasing algorithm bias. Hence, creating a gender-neutral job description using words like individual instead of man or woman is crucial.
Increase the visibility of female role models: to encourage more women in the AI sector; it is essential to establish female role models by promoting their achievements and success stories which can motivate other women to consider a career in technology. Besides this, the company should also ensure that its diversity, equity, and inclusion strategy extend to technical roles.
We must ensure that AI doesn’t carry forward existing gender bias in society from the past decade. So, we need to create awareness among related stakeholders involved in the AI process about how bias data can negatively impact the decision against a particular class of society. We can take several concrete steps to reduce/remove such biases by involving women in AI and using robust controls, i.e. Seclea Platform, to prevent biases in society. Overall, gender bias has a more significant influence on normative and societal principles, so it needs to be addressed by creating a more democratic, inclusive, and equitable digital economy for everyone.
What next?
Gender inequality in AI solutions is a significant issue and risk for your organisation. All AI regulations and frameworks require fairness and non-discrimination. So, to ensure your AI project provides the desired benefits to your organisation, make sure your AI is fair.
Seclea provides tools for your AI stakeholders (Data Scientists, Ethics Leads, Risk & Compliance Managers) to ensure fair and transparent AI solutions that meet relevant AI regulations. We are here to help; email us at hello@seclea.com or fill out the short form.
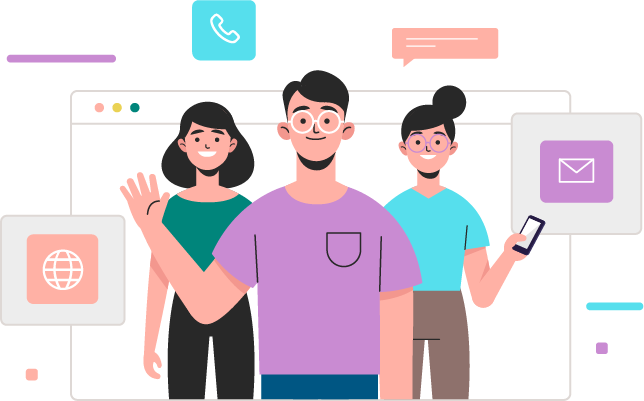