Societal bias in AI: A growing concern and possible solutions
Artificial intelligence (AI) is a technology that enables machines to work with human-like intelligence, as seen in applications like facial recognition, medical diagnosis, and self-driving cars. AI has significantly improved efficiency, reduced the need for human involvement in repetitive tasks, and enabled creative work. Additionally, AI has eased day-to-day life through intelligent home devices, smartphones, and smart cars. As AI becomes an integral part of our lives, it makes critical banking, recruiting, healthcare, and criminal justice decisions, directly impacting human lives. AI is creating new momentum by combining human and machine capabilities to address complex decisions. However, there have been numerous cases where AI has failed to make fair decisions, leading to biased outcomes such as candidate filtering, loan provision, or medical diagnoses. This is commonly referred to as AI bias.
Scope of the societal bias
Inherent prejudices cause AI bias in the data used to train models, leading to social discrimination and a lack of opportunities. Bias can seep into data primarily due to preferences or exclusions in training data, how information is obtained, how algorithms are designed, and how AI outputs are interpreted. Societal bias is a pattern that reflects unfair or incorrect social assumptions and judgments, often due to social intolerance or institutional discrimination. Societal bias includes discrimination based on race, gender, biological sex, age, and culture. Here are a few examples of existing social biases in AI across various sectors:
Health sector: The health sector is where human bias embedded in AI can lead to significant issues, as misdiagnosing or incorrect decisions could cost lives. A study published in the Journal of Emergency Medicine in 2020 highlighted that black patients were 40% less likely to receive pain medication in U.S. emergency departments than white patients due to social bias caused by racial, ethnic, and class stereotypes. Another article in The Economist in 2021 stated that pulse oximeters, which measure blood oxygen saturation through the skin, were found to be biased against non-white patients by overestimating oxygen saturation.
Finance sector: AI bias in the financial industry can disproportionately reward certain groups and be unfair to others based on gender, sex, colour, or ethnicity. A study from Stanford University’s Graduate School of Business (2021) found that credit scoring models were 5 to 10% less accurate for individuals with higher incomes and non-minority backgrounds. These models are biased against disadvantaged borrowers with little credit history, leading to automatically low credit scores.
Educational sector: AI is increasingly used in colleges and universities for admissions, advising, courseware, and assessment. In 2020, the University of Texas employed an algorithm to evaluate applicants for its PhD program, but the algorithm reduced opportunities for applicants from diverse backgrounds. In 2021, The Markup investigated the advising software Navigate, used by large public universities for consulting EAB, and found that black students were at a higher risk of not completing their bachelor’s degree than white students.
Criminal justice: AI tools are now used for prevention, protection, and solving cases. One of the most popular tools for detecting crime is facial recognition. However, due to the system’s poor performance, an innocent person was arrested based on false facial recognition, as the New York Times reported in 2020.
Social Media: Social media platforms are popular modern tools for people to express their views and share ideas. One of the most popular platforms, Twitter, apologised for its cropping algorithm’s bias toward black faces.
Skewed data with less representation of certain groups is one of the leading causes of societal AI bias. This bias violates fundamental human rights and perpetuates human error by excluding individuals from participating in social and economic activities. Scholars have highlighted how these automated decisions could deprive people of government benefits, discriminate based on sex, skin colour, age, and other forms of difference, and influence decisions on who should be set free, imprisoned, or targeted for economic exploitation. Most importantly, it undermines the potential of AI for businesses and society by fostering mistrust and producing distorted results.
How to address the AI bias?
So, how can we address societal bias to create a fairer society?
- Design for inclusive models: Involve humanists and social scientists in model development to ensure that AI models do not inherit biases in human judgment. If risks are identified in AI models, set measurable goals to mitigate them and ensure equal performance across diverse groups.
- Mitigate bias before, during, and after modelling: Ensuring fairness in algorithms is crucial, so using diverse and representative input data is essential. Ensure that all protected/sensitive class groups have predictive equality for false positive and false negative rates.
- Testing: Testing is essential for ensuring unbiased data, including model performance in complex situations. Continuously retest models with real-life data and gather user feedback to help reduce bias.
- Explainable AI: Understanding AI’s processes and prediction outputs is crucial for identifying and mitigating bias in data or algorithms. Therefore, it is necessary to develop explainable and interpretable AI to help organisations identify deep biases that can arise due to complex algorithms.
- Use available AI bias-detecting tools: IBM, Google, and Microsoft have developed tools to detect and mitigate bias in data. Google’s AI Fairness 360, and Microsoft’s Fairlearn toolkit are examples of tools to reduce bias in data and models. Seclea Platform has a state-of-the-art toolkit for bias detection and mitigation.
- Follow legal obligations: The General Data Protection Regulation (GDPR) and EU Artificial Intelligence Act (AIA) mandate the prevention of discriminatory effects when using personal data by employing appropriate mathematical or statistical procedures to ensure the risk of error is minimised and to prevent discriminatory impacts based on race, political opinions, religion, health, or sexual orientation. Therefore, complying with the existing GDPR and upcoming EU AIA is essential for making fair decisions in AI.
Ensuring responsible AI requires technical and institutional approaches to control and monitor societal biases. This involves continuous training for stakeholders involved in building AI technology, such as data scientists, developers, and decision-makers. Reducing societal discrimination requires evaluating data and algorithms and following best practices while designing and utilising algorithms. By addressing societal biases in AI, we can foster more equitable systems and promote trust in AI-driven decisions, ultimately benefiting businesses and society.
Addressing societal biases is paramount as we integrate AI into our society. By understanding the potential consequences of AI-driven decisions and taking proactive measures to eliminate biases, we can create more inclusive and equitable systems that improve the lives of individuals and communities.
To further support these efforts, governments, organisations, and educational institutions should invest in research and development to create innovative solutions for addressing bias in AI systems. This includes interdisciplinary collaboration between computer scientists, social scientists, and ethicists to ensure a comprehensive understanding of the various dimensions of bias.
Raising awareness about AI bias among the public and stakeholders involved in AI development is crucial. This can be achieved through educational programs, workshops, and conferences addressing AI’s ethical implications and promoting responsible AI practices.
Organisations should also adopt transparent and accountable AI governance frameworks that include guidelines and principles to ensure the ethical use of AI technologies. By implementing such frameworks, organisations can demonstrate their commitment to responsible AI development and enhance trust among users and stakeholders.
Addressing societal bias in AI is a collective effort that requires the active participation of governments, organisations, researchers, developers, and users. By working together, we can create a more just and equitable society that harnesses the power of AI for the greater good.
In conclusion, the key to reducing societal bias in AI lies in understanding the sources of bias, designing inclusive models, continuously testing and refining algorithms, promoting transparency and explainability, using available tools to detect and mitigate bias, and adhering to legal obligations. By adopting these best practices, we can harness the power of AI to promote fairness and equality, fostering trust in AI-driven technologies and maximising their potential benefits for businesses and society.
Building a Better World with Artificial Intelligence!
To mitigate AI bias, organisations must adopt comprehensive governance policies that outline responsible AI principles and include mechanisms to enforce and monitor compliance throughout the AI lifecycle.
Seclea provides tools designed for AI stakeholders, such as Data Scientists, Ethics Leads, and Risk & Compliance Managers, to enforce, monitor, and achieve compliance with governance policies at every stage of the AI lifecycle – from development to deployment activities. Our solutions offer real-time monitoring and reporting, ensuring that AI systems adhere to ethical guidelines and responsible AI practices.
We help you implement and maintain responsible AI in your organisation. To learn more about our services and how we can support you, please email us at hello@seclea.com or fill out the short form on our website. Together, we can work towards creating AI systems that promote fairness, transparency, and accountability, fostering trust and maximising the potential benefits of AI for businesses and society.
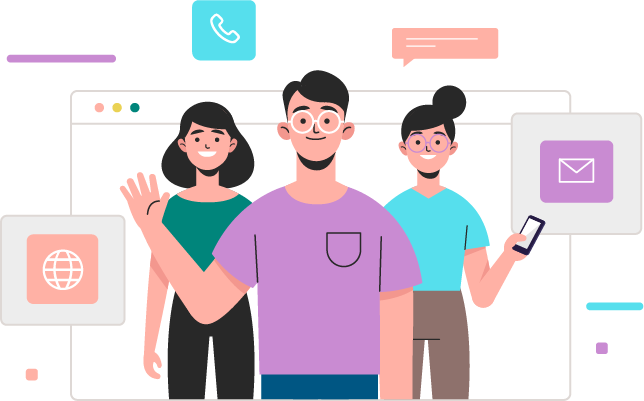