Artificial intelligence (AI) will integrate into almost all the devices and services humans interact with, from home appliances to industrial control systems. With underlying benefits of AI being cheaper, faster, and more efficient than humans. However, though AI has multiple uses, we can’t ignore its risks, including privacy violations, discrimination, and the inability to explain and audit AI applications. In addition, AI often deals with personal, including sensitive information, and relies on the machine to decide, which means the device can easily overlook the issue of fairness, responsibility, and respect for human rights. Thus, we need responsible AI to overcome challenges. Responsible AI refers to the practice of designing, developing and deploying AI which is fair and trustworthy. The Responsible AI principles are as follows such as:
- Fairness: is a core principle of responsible AI that identifies any biases and unfairness in AI applications. Fairness is the absence of unequal treatment based on gender, race, religion, colour and age. As biases could be in the data or algorithms or any historical biases perpetuated in AI applications Thus, Fairness in AI can achieve through various available bias detection tools such as the seclea platform to examine biases in the data and algorithm.
- Transparency: explaining machine learning models and decisions making processes in an understandable way for its user. Transparency is one of the essential principles for making responsible AI. It enables people to understand how the AI system is developed, trained, operated and deployed in a particular business so that consumers can make an appropriate choice based on the information they receive. Transparency can be achieved by using a simpler model rather than a complex one, modifying the inputs, and designing the model user-friendly. Such practice can identify any risks or biases possessed by the AI system.
- Accountability: one of the crucial principles of responsible AI is accountability which helps identify the biases in AI applications and makes user companies accountable for the damage. Further, each stakeholder building the AI application should also be liable if the AI system goes wrong and make them responsible for their actions. For example, if a self-driving car’s algorithm directs the car and meets with an accident, who is accountable for the accident? Also, during the evaluation of AI applications, users should clearly explain both the positive and negative consequences of AI applications.
- Explanation: enables AI-based decisions and actions for people affected by AI system outcomes. It can be achieved in different ways. Researchers have identified explainability as a requirement for AI clinical decision support systems as the responsibility of doctors to explain the result to the patient to make an informed decision. Such practice can build AI trustable and responsible.
- Reliability & Safety: Another crucial principle of responsible AI is reliability and safety. This principle ensures that AI applications behave rationally and understandably, verify results and ensure safety. Besides that, it is vital to check whether the model performance is consistent in multiple scenarios.
- Privacy & Security: Privacy and security are the most important parts of responsible AI, including data privacy and security. As per GDPR, AI systems must comply with privacy laws requiring transparency about the data collection, storage and uses of such data. For instance, the Cambridge Analytica scandal, where Facebook users’ data uses without consent, caused distrust and reputational damage to the company. Thus, Privacy and security play essential aspects in making AI responsible.
- AI Risk & Compliance Management: AI risk management helps to identify, monitor and manage the potential AI risks. Compliance ensures that the company operates within legal and ethical boundaries while collecting, dealing and using the data. With AI applications, detailed analysis of all types of risks, analysis and feedback help the company achieve the goal in a cost-effective, flexible and timely manner. For instance, using AI applications in the financial sector helps capture, filter and analyse data and solve the false positives banks face.
To translate the principle into practice, government, organisations, and individuals can contribute to making AI responsible. So, here are a few examples of how each stakeholder can play a significant role in following the principles of AI.
- Government: The government needs to introduce regulation specifically for the use of AI, which includes effectively protecting human rights and prohibiting technology from violating such rights. Encourage companies by recognising their work and providing the support they need.
- Organisation: As an organisation, AI assessment should carry out at each point of the life cycle of AI systems, including design, development, deployment, and monitoring of outcomes and impacts. Besides that, continued testing of the application and taking feedback from the stakeholder reduces the bias.
- Companies should protect the most vulnerable by setting up a system that allows people AI systems have harmed to make a complaint and get compensation.
- Introducing an audit in a company by documenting the development of the model in a company’s early stage of model deployment. Further clear evolution benchmarks and metrics need to be placed. So that both agency and third-party evaluators can identify whether the company delivering outcomes meets the code of conduct, such steps set a good standard practice in a company by preventing risk and reputational damage and leading towards responsible AI.
- Individual: A company’s individual (data scientist, data engineer, or programmer) can ensure the implementation of principles by following the company guidelines, checking the data, building fair models, and documenting all the steps. Besides, while making any model, it needs to be tested multiple times. An individual must take responsibility for ensuring fairness in the AI life cycle and hold the blame if they fail to create fair models.
Having principles alone can’t make AI responsible. Instead, the key stakeholders need to understand and appeal to implementation in AI systems. Further, every organisation using it should know the importance of implementing responsible AI principles. So government, organisations and individuals need to develop effective plans of action to implement existing principles for making AI accountable.
What next?
Seclea provides tools for your AI stakeholders (Data Scientists, Ethics Leads, Risk & Compliance Managers) to ensure Responsible AI policies are integrated into the AI development and deployment activities and real-time monitoring and reporting. We are here to help; email us at hello@seclea.com or fill out the short form.
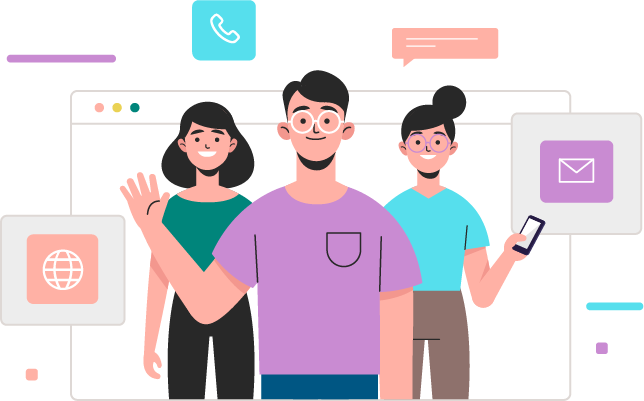