What is explainable AI?
Explainable AI (XAI) refers to a set of processes and methods which allows human users to comprehend and trust the result created by the machine learning algorithm. The Royal Society emphasises this as a system focusing on different explainability needs per context. For instance, the explanations given to technical and non-technical people are different. So, the XAI definition depends upon the context and user, which helps people understand the machine’s decision-making process. The main objective of XAI is to enable humans to understand, trust and effectively manage the AI’s prediction accuracy. XAI is critical for sensitive applications such as clinical decision-making or financial services.
Why is Explainable AI important?
AI applications for consumer and regulation-sensitive, such as self-driving cars, the healthcare sector, the financial industry and autonomous weapons, can negatively affect human lives. As machines don’t have self-judgement, it is crucial to understand the decision-making process. There were various incidences of poor judgement of machine-led biases and risk in the past. For instance, an autopilot self-driving car hit and killed a pedestrian. Similarly, Apple credit cards were biased against women. In addition, the AI application in the health sector possesses bias toward black and minority groups. Likewise, AI hiring tools such as LinkedIn’s AI recommended more men than women for jobs by learning the behaviour and patterns of job skills and search. The pew research poll found that AI biases are more likely to affect disadvantaged groups already poor and vulnerable. Therefore, it could decrease the credibility of AI, building distrust of them.
So, explainable AI helps to give a complete understanding of how and, more importantly, why an AI make decisions in specific ways.
What are the challenges of explainable AI?
The study on the principles and practice of explainable machine learning provides four suggestions for creating explainability, including explanation by simplification, describing the contribution of each feature and using graphical visualisation. However, the studies pointed out that simplification might not be easy, features could be interrelated, the explanation might only be able to explain the instances, and graphic visualisation might not always be accurate due to the quality and sources of the data. Similarly, the study on the perils and pitfalls of explainable AI presents challenges.
Lack of expertise: One of the significant challenges explainable AI faces is the lack of experts. It is challenging to generate a meaningful explanation for a model’s decision. Further, it hinders the identification of whether the decisions are fair or not.
Inherent Bias: Most datasets collected from various data sources are often biased and can directly impact the model’s decision. Further, as the decisions are based on the complex model, even the translator who explains the model can have inherently limited choices that can impact the result.
Changing algorithm: The algorithm is not static and learns its own decision. Hence, algorithms are dynamic and change over time. So, it isn’t easy for explainable AI to identify whether the AI is fair to use or not with the same explanation over time. The more dynamic the algorithm is, the more challenging the XAI.
Context dependency: It is also essential to understand that everyone is different, so XAI decisions can’t be generalised to all. When deciding on an individual explainable AI, it is difficult to explain why the particular decision differed from others.
Causality: Though statical techniques applied to data can show a good correlation between inputs and outputs, it doesn’t mean it is true. The AI model is complex and challenging to verify whether the explained model is correct or not? Also, the explanation of causality might change over time.
How do we overcome the Explainable AI challenges?
Using global and local explanations, XAI can be interpreted. The global surrogate model is an interpretable model that approximates the prediction of the black-box model by using linear and decision tree models—the global surrogate on the R-square between features. The global surrogate model approach is intuitive and straightforward, which helps make it easier to explain. Similarly, Local explanations can explain a single prediction, giving the prediction one at a time. For instance, if a bank rejects a person’s loan by using the local explanation view to explain the reason for rejection. The local model adopts two models: local surrogates and counterfactual models. A local surrogate model can explain a single prediction for not being able to pass the loan. In counterfactual explanation, the model defines minimal changes to the features of the instance of the interest, so its prediction changes to the desired one. Further, it provides feedback for approving the loan.
Potential pitfalls of Explainable AI!
Explanation of bad decisions doesn’t make the decision reasonable. Along with developing AI applications whose behaviour and decisions can be clearly explained, we need to make sure that the AI application is also fair, transparent, accountable, auditable and continuously monitored—making sure that an AI application is a responsible technology whose objective is to provide better and fairer services to its users. Explainable AI is a good start, but organisations must expand it to responsible, accountable and regulatory compliance AI paradigms. The fear is that organisations deploy XAI and consider their work done. This is a risky option as the dynamic nature of AI and factors related to AI fairness, accountability, and transparency can have a negative impact on the organisation’s business operations and brand image.
Explainable AI is one of many components organisations must put in place for a successful and safe deployment and usage of AI applications. But on its own, it is not the complete solution and can potentially lead to undesirable consequences.
Conclusion
However, explainable AI alone is not enough for making accurate decisions. The simplicity of the inherently explainable AI model seems appealing. However, such an explanation might miss the presence of other issues. Besides that, the increase in transparency can hamper users’ ability to detect sizable model errors and correct them due to an overload of information. Similarly, the methods used for explainable AI, such as LIME (Local Interpretable Model Agnostic Explanation) and SHAP (Shapley Additive exPlanations), are generic for making explanations. Data and algorithms are dynamic and need a more detailed explanation than a static and generic answer. So, it is vital for explainable AI not just to justify the answer but provide a reliable and evidence-based answer that can ensure trust within AI. Besides, XAI helps troubleshoot and systems audit to improve the model performance and identify biases of AI.
What next?
Explainable AI helps organisations understand and manage their AI solutions better. It also de-risks AI adoption in heavily regulated industries, and having explainable AI is one of the core requirements for all AI regulations and frameworks. Combining Explainable AI with AI fairness, transparency, and accountability ensures that your AI applications are responsible and regulatory compliant.
Seclea provides tools for your AI stakeholders (Data Scientists, Ethics Leads, Risk & Compliance Managers) to develop and deploy responsible AI solutions that meet relevant AI regulations. We are here to help; email us at hello@seclea.com or fill out the short form.
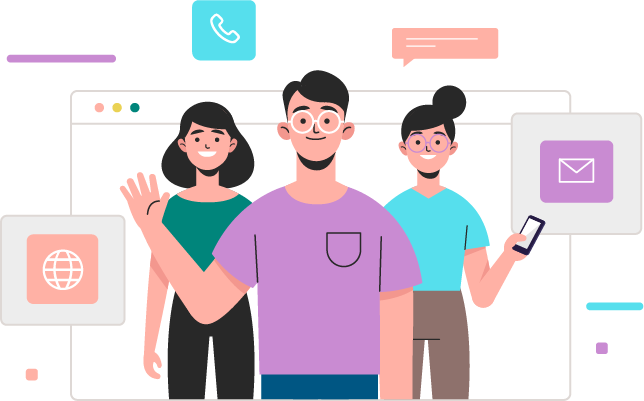