What is Explainable AI?
Explainable AI (XAI) refers to methods and techniques that make an AI application’s behaviour and decisions understandable to humans. According to the definition by IBM, “Explainable AI” is a set of processes and methods that allow humans to understand and trust the results and outputs created by machine learning models. XAI helps to identify the strengths and weaknesses of the decision-making model. Further, it determines if there are any biases or risks possessed by AI or not. In simple terms, XAI enables a straightforward interpretation of AI applications’ behaviour and decisions.
AI use in Financial Services!
AI’s adoption in the financial sector is growing. Nearly 60% of financial services companies use at least one AI application, from dealing with customers to fraud detection. Similarly, an Open text survey found that 80% of banks recognise the benefits of AI, and 75% have already started using it. According to a report on the impact of AI in the banking sector, 56% use AI for risk management and 52% for revenue generation in the banking sector. Banks are projected to save approximately $447 billion using AI applications by 2023. Banks use AI for 24/7 customer service and securing their business from fraud detection and efficient management of credit/trading risks. Here are a few advantages of using AI in the financial sector, such as:
- Chatbots. They work 24/7 and address customer queries, saving bank time and expenses. For instance, the Commonwealth Bank in Australia uses artificial intelligence Ceba chatbots to assist customers with over 200 banking tasks.
- Risk management: One of the most critical areas in which AI applications are used in banking includes credit risk, market risk, operational risk, and cyber security risk. To counter these problems, the banking sector uses AI platforms. Using AI applications, the banking sector predicts house prices, alerts on non-legit transactions, and provides a credit score for a credit card.
- Transaction data enrichment: AI helps convert transactions to readable text, allowing banks and customers to understand where they spent money and with whom. Such practices can prevent non-legit transactions and alert banks from preventing anti-fraud activities like money laundering.
- Data Security: Another critical area for AI applications is data security. According to the Federal Trade Commission report for 2020, 2.2 million consumers were reported as victims of fraud. Using AI applications helps programmes analyse customer behaviour, location, and habits and notify the bank of any unusual transaction. This would enable the identification of the account and prevent fraud.
Benefits of Explainable AI in the Financial Sector?
According to Gartner, by 2025, 30% of government and large enterprise contracts to purchase AI products and services will require the use of explainable and ethical AI. Using AI applications has helped the financial sector by increasing productivity and reducing errors through a faster decision-making process. However, there are a few challenges in implementing AI in the financial industry, complex algorithms used in the finance sector make it difficult to understand the decision-making process, making it more vulnerable to risks and biases, especially when regulators require AI application’s behaviour and decisions to be fully explainable.
Explainable AI will play a significant role in the financial sector as banks cannot have a situation where they cannot explain why they couldn’t lend credit. An explainable AI model can help overcome the black box issues and inability to explain the behaviour and decisions of an AI application. The XAI allows banks to build transparency and trust in AI applications through intuitive and understandable interfaces for human users with better accuracy. Furthermore, XAI has become a banking regulation by ensuring that bank employees also understand the outcome produced by the AI application.
Furthermore, the industry needs to effectively run the complex model in the financial sector to keep humans in the loop to avoid major accidents. All stakeholders building AI applications should understand and prioritise explainability according to a user-centric approach. A human in the loop is ineffective if the human cannot understand the behaviour and decisions of the respective AI application. Such an approach reinforces the need for AI explainability.
In the financial sector, XAI can help to boost businesses by assisting them in understanding the decision-making process, which will help to build trust in AI and make them responsible. The financial sector needs to provide evidence for clients, especially for a credit card or mortgage approval. Besides, that company can identify and manage the risk through faster and more consistent technology adoption. As a result, XAI helps to build trustable AI applications, which reduces bias and leads to greater efficiencies for companies. Also, explainable AI can bridge the gap between understanding the AI’s financial decisions and customers.
How to implement Explainable AI in a Financial Services Application?
Blackbox AI solutions can pose a significant issue and risk for your organisation. All AI regulations and frameworks require financial services organisations to explain this AI application’s behaviour and decisions. So, to ensure your AI project provides the desired benefits to your organisation, ensure your AI is explainable.
Seclea provides tools for Data Scientists to ensure AI application’s decisions, behaviour, and evolution can be explained with traceability. We are here to help; email us at hello@seclea.com or fill out the short form.
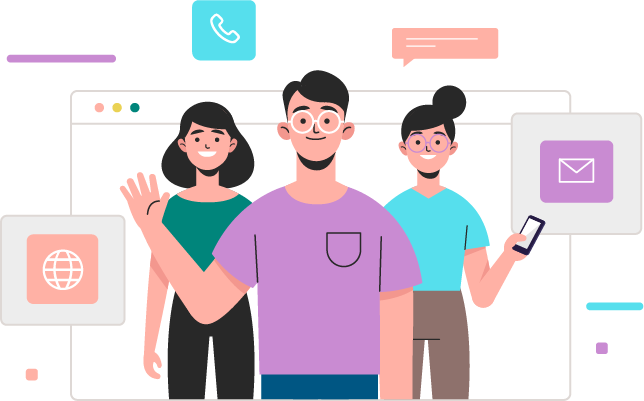