In a nutshell, AI replicates human analytical and decision-making capabilities. And it is increasingly being integrated into various systems that can have a direct physical and psychological impact on humans, such as self-driving cars, smart devices, and automated finance. AI can potentially be classified into three categories from the so-called perspective of ‘how intelligent they are:
- Narrow/Weak AI: Narrow/Weak AI is goal-oriented, designed to perform well-defined tasks such as facial recognition, spam email filter, self-driving car, speech recognition etc. Narrow AI doesn’t mimic or replicate human intelligence but simulates human behaviour based on a narrow range of parameters and contexts.
- Strong/Deep AI: Strong AI or deep AI refers to mimicking human intelligence with the ability to learn and apply human-level intelligence or behaviour to resolve problems. Strong/deep AI trains machines to replicate the functionality and flexibility of the human brain. However, it’s challenging to replicate because of the lack of comprehensive function of the human brain. Examples in the future can be general-purpose AI assistants, household general-purpose robots, etc.; the generality of AI and its capabilities is the key difference compared to weak/narrow AI.
- Super Intelligence: Super Intelligence goes beyond mimicking human intelligence – surpassing the capacity of human intelligence. Super intelligence’s decision-making and problem-solving capabilities would be far superior to humans. Though it sounds appealing, it comes with unknown consequences with potentially severe impacts on humanity or survival.
AI’s rapid growth has raised concerns about it and its potential impact on human life. AI misconduct and unfair outcomes based on race; gender are a few examples of how so-called intelligent technology can go wrong. Elon Musk, CEO of SpaceX, pointed out that AI could be dangerous as it can do everything better than us and Bill Gates agreed about AI’s possible threats. Stephen Hawking predicted that AI could “spell the end of the human race“. A few examples of how AI could potentially be risky to our society are:
- Fully autonomous weapon: One of the examples of how advances in machines could lead to risk is a fully autonomous weapon. The machine could select and fire independently on its target. This is commonly referred to as a killer robot. It would be a dangerous world if machines decided and potentially caused the destruction of humans.
- Black Box model: It is essential to understand how the machine works and how it makes decisions that affect human lives. Applications like approving a loan, processing the CVs of candidates, or diagnosing a disease – decisions made by these applications can have long-reaching consequences for individuals. According to MIT professor Tommi Jaakkola, the Black box model is causing problems now and will continue in future. It is impossible to identify when the machine goes wrong and affects human life with such an existing black-box model.
- Bias in AI: When an algorithm produces systemically prejudiced results due to erroneous assumptions in the machine learning process, it may consciously or unconsciously affect an individual or a group. Bias could be either cognitive prejudicial bias or a lack of a balanced dataset for modelling. Like algorithms built with US healthcare data being biased against black people, Amazon’s algorithms being biased against women and class imbalance being a leading issue in facial recognition software are a few examples of existing biases in AI.
- Invasion of privacy: Most companies collect private data like age, location and preference for their benefit. Algorithms, recommendation engines, and each network amplify the ability to use sensitive personal information without a person’s consent. For example, in China, the social credit system records the activity and gives a score accordingly. So, AI systems based on such data can violate human rights.
We can avoid such problems by making AI fair, transparent and accountable through Responsible AI practices.
“Responsible AI ensures that the process of designing, developing, and deploying AI has clear alignment with our social, ethical and corporate values to foster trust between humans and machines.”
The main objective of explainable AI is to interpret a model that can explain how it makes predictions, such as why your application for a mortgage or diagnosis with cancer was rejected. However, there are still trust issues with AI, as a study from Accenture’s 2022 tech vision found that 35% of global consumers trust AI implemented in organisations, whereas 77% think it’s misused.
Responsible AI‘s scope is significantly more extensive than explainable AI. Responsible AI encompasses AI transparency, fairness, privacy, interpretability and security. So, to address the AI trust issue, we can use Responsible AI practices in each of the following aspects:
- Algorithms and dataset: Examine the training data to ensure the data’s diversity, quality, and quantity. Assess and monitor model performance both during training and in production.
- Risk mitigation: It plays a vital role in making AI Responsible. AI presents numerous challenges for both organisations and individuals. These challenges translate into substantial risks for all AI stakeholders. For example, AI bias is a real AI risk that has severe implications for underrepresented segments of our society. To effectively identify such threats, manage them and track them throughout the lifecycle of an AI application is crucial. An effective Responsible AI programme will work and mitigate AI risks for all AI stakeholders.
- Establishing techniques to prevent bias: It is crucial to develop strategies in a company that helps to identify bias at the technical, operational and organisational level. For example, the technical story involves developing tools to identify the potential source and impact of the discrimination. Similar operational levels include improving or training the data collection teams or conducting a third-party evaluation to ensure fairness. Responsible AI entrenches AI transparency and accountability to reduce AI bias in data, design and training.
- Making AI Accountable: There should be a mechanism to control automated applications with human involvement by promoting accountability. For example, when humans are in the loop for taking decisions, using fully autonomous weapons can make humans accountable for their actions rather than robots. There could be a risk of escaping liability for the harm caused by a gun that acted independently and produced a malfunctioning product. Extending the accountability further, Responsible AI can enforce AI accountability to data sources used for training, data scientists/engineers, and AI project managers for AI algorithm design and development and human operators.
The future of the coming generation depends on how we regulate automated machines—global efforts on both regulations and standards to ensure well-defined and managed AI frameworks. To build risk-free and fair AI applications, each organisation needs to develop a Responsible AI policy that considers the relevant regulations, standards, and the company’s own corporate responsibility policies. By enforcing an organisation’s level of Responsible AI policies, we can ensure that AI innovation is for the betterment of society and does not pose a risk to the organisation. We need to focus on building Responsible AI to make an intelligent and fairer society.
What next?
Organisations need to adopt Responsible AI principles and, based on these principles, develop a policy along with mechanisms to enforce and monitor adherence.
Seclea provides tools for your AI stakeholders (Data Scientists, Ethics Leads, Risk & Compliance Managers) to ensure Responsible AI policies are integrated into the AI development and deployment activities and real-time monitoring and reporting. We are here to help; email us at hello@seclea.com or fill out the short form.
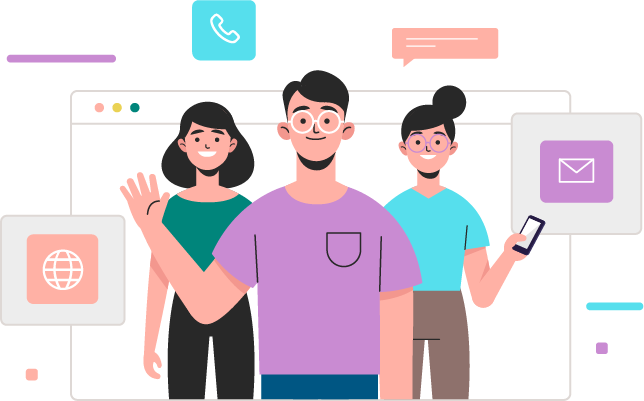